29. April 2015
The Influence of Indirect Ties on Social Network Dynamics
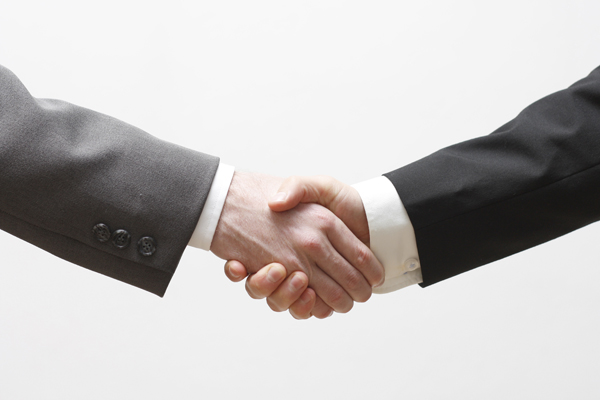
How are connections made and messages shared in social networks?
Direct (i.e., inter-personal) social ties have been intensely studied in the context of computer-mediated social networks such as Facebook, Twitter, etc. Yet in real life, we often rely on friends of our friends for recommendations (of doctors, schools, or babysitters), for introduction to a new job opportunity, and for many other occasional needs. However, such indirect ties (e.g., friends of friends or 2-hop ties) have been less studied in the past.
Homophily and Triadic Closure
Sociology provides two theories closely related to the properties of indirect ties: homophily and triadic closure.
First, the theory of homophily postulates that people tend to form ties with others who have similar characteristics. Moreover, a stronger relationship implies greater similarity. Second, the principle of triadic closure, proposed by M. Granovetter, states that two individuals with a common friend are likely to become friends in the near future.
In particular, according to Granovetter’s idea of a forbidden triad, a triad of (possible) ties between three individuals, Alice, Bob and Charlie (Figure 1), in which there are strong ties between Alice and Bob, and between Bob and Charlie, but no tie between Alice and Charlie, is unlikely to exist. When it does, according to the theory of triadic closure, it is typically quickly closed with the formation of a tie between Alice and Charlie. Intuitively, these two will meet at some point as they have a common friend, Bob. The triadic closure has been demonstrated as a fundamental principle for social network dynamics.
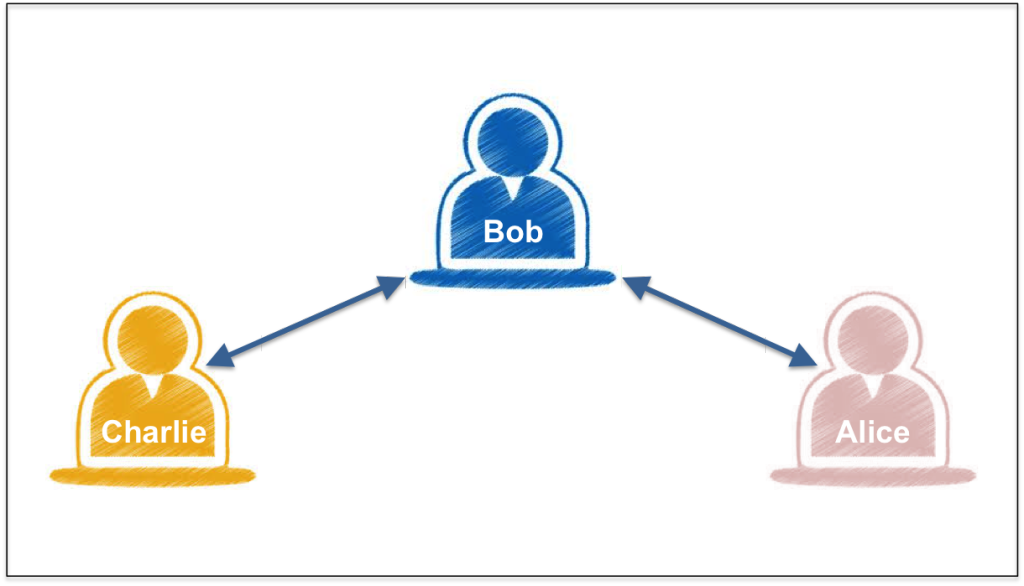
Figure 1: The forbidden triad
Lately, large online social networks provided unprecedented opportunities to study dynamics of networks. Thus, many studies examined the evolution of groups or analyzed membership and relationship dynamics in these networks. We studied these properties on real social networks of gamers, conference attendees and co-authorships and examined how they associate with the dynamics of each network with respect to the formation of new ties between two individuals and diffusion of new information in each network.
Formation of New Ties
The basic problem of tie formation prediction revolves around the idea of predicting when a tie will form between two non-connected individuals. Intuitively, the further they are in the network, and the less information we have about their interactions makes this prediction task more difficult.
To this end, we studied indirect ties between individuals and how they can improve the performance of this prediction task. We found that indirect ties carry a significant power in predicting when new ties will form between two individuals, especially when we utilize information such as the number of indirect ties connecting the two individuals and also the strength of the relationship between each of them and their common friend(s). Moreover, we found that the implicit strength of an indirect tie positively correlates with the speed with which a new tie is formed, and further, that this newly connected pair of individuals will have more interactions when this implicit strength is higher. Also important is that this prediction power remains fairly high even when we consider 3-hop indirect ties: that is, friends-of-friends-of-friends!
Diffusion of New Information
Diffusion of new information is a fundamental process in social networks and has been extensively studied in the past. In fact, some studies have shown that the evolution of a network is affected by the diffusion of information in the network and vice versa. However, diffusion of information has been typically studied in a step-wise fashion, that is, how new information will propagate in the network step-by-step, or from individual to individual.
Indeed, it would be ideal to predict how new information will diffuse in the network much earlier in advance. To this end, we studied how indirect ties can be used in this diffusion prediction task. That is, given that an individual received a piece of information at time step T, we investigated which other individuals are more likely to receive this information at time step T+2 or T+3, given information about the indirect ties of individuals. We found that the strength of indirect ties (i.e., ties non-formed yet) can predict well diffusion paths between distant individuals in the network. That is, indirect paths formed with strong ties (e.g., path 1 in Figure 2) lead to more diffusion than weak indirect paths (e.g., path 2 in Figure 2).
These findings can be applied in various domains. For example, it can be used by disease control agencies around the world when they are trying to stop a deadly virus from spreading out of control. It can also be very helpful to online social network (OSN) providers who can customize strategies for preventing or accelerating information spreading. For example, to contain malware, OSN providers could block related messages sent to susceptible individuals several time steps before the malware arrives, or disseminate official patches in advance. Similarly, marketers could accelerate their advertisements spreading in the network by discovering who will be the next susceptible to buy a product, or who they should contact first to disseminate important advertisement messages.
Photo Credit:
The feature image is taken from the Flickr-Account of 드림포유 and is usabel under creative commons license. Find the Handshake on Flickr.
This article is based on the original paper “The Influence of Indirect Ties on Social Network Dynamics”, published in the 6th International Conference on Social Informatics, 2014. Authors of original paper: Xiang Zuo (1), Jeremy Blackburn (2), Nicolas Kourtellis (3), John Skvoretz (4), and Adriana Iamnitchi (1). (1) Department of Computer Science & Engineering, University of South Florida, FL, USA. (2) Telefonica Research, Barcelona, Spain. (3) Yahoo Labs, Barcelona, Spain. (4) Department of Sociology, University of South Florida, FL, USA.